A Bayesian machine scientist to aid in the solution of challenging scientific problems
Times cited: 125
Guimera, R, Reichardt, I, Aguilar-Mogas, A, Massucci, FA, Miranda, M, Pallares, J, Sales-Pardo, M.
Sci. Adv.
6 (5)
,
eaav6971
(2020).
Closed-form, interpretable mathematical models have been instrumental for advancing our understanding of the world; with the data revolution, we may now be in a position to uncover new such models for many systems from physics to the social sciences. However, to deal with increasing amounts of data, we need “machine scientists” that are able to extract these models automatically from data. Here, we introduce a Bayesian machine scientist, which establishes the plausibility of models using explicit approximations to the exact marginal posterior over models and establishes its prior expectations about models by learning from a large empirical corpus of mathematical expressions. It explores the space of models using Markov chain Monte Carlo. We show that this approach uncovers accurate models for synthetic and real data and provides out-of-sample predictions that are more accurate than those of existing approaches and of other nonparametric methods.
Media coverage
Related downloads
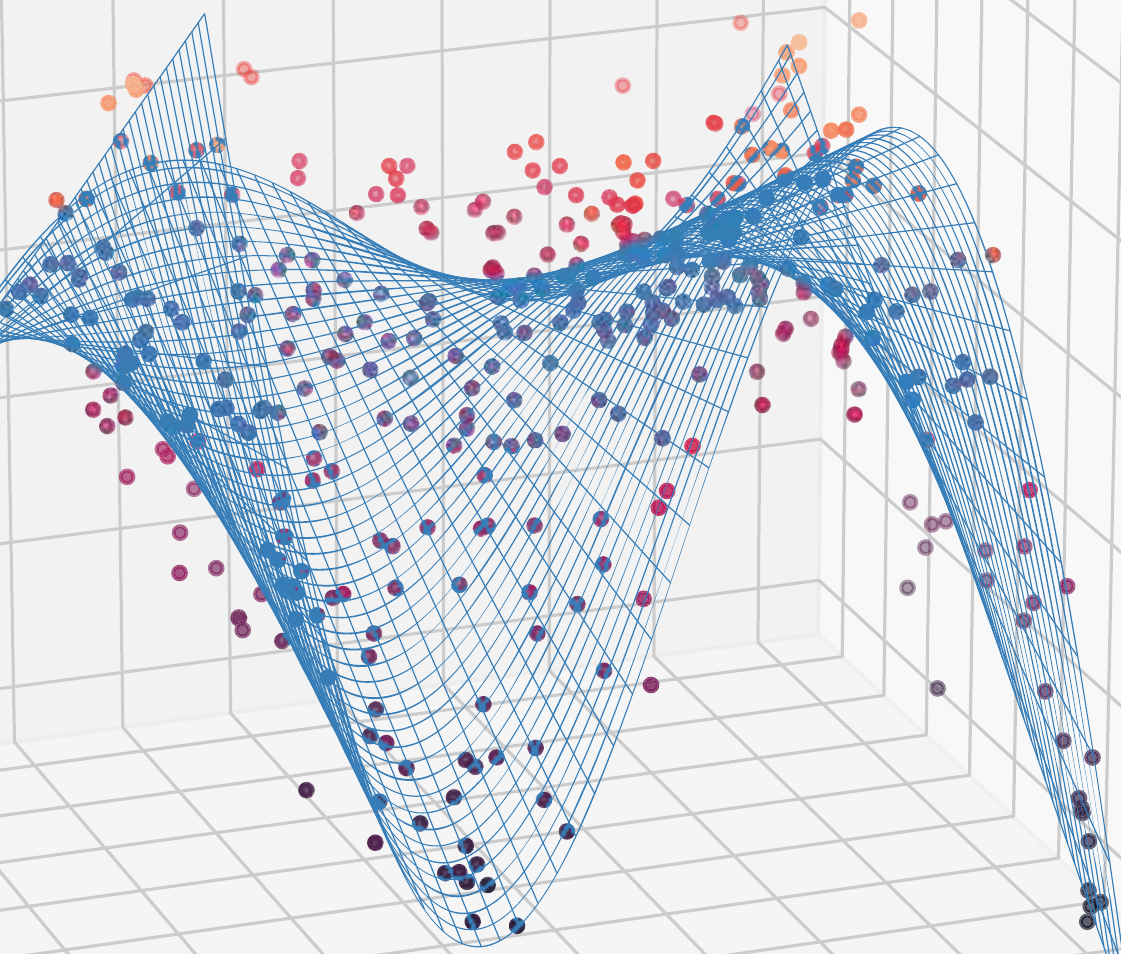